The big breakthroughs and new companies that are going to be created in the future are interdisciplinary ones, where you make connections between two disparate subjects, and that’s going to happen again and again in the next 20 years. It’s going to be where a lot of the big breakthroughs come from.
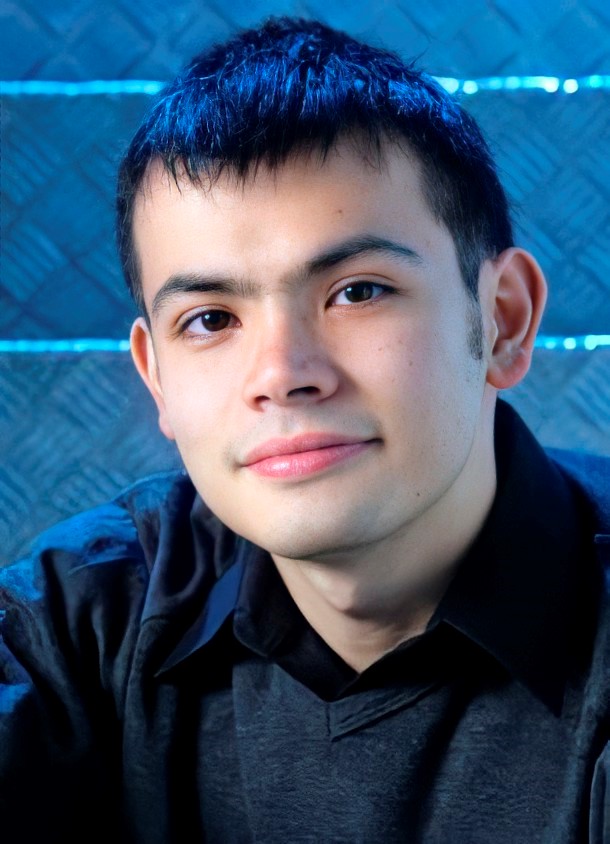
Demis Hassabis was born in London, England. He is of both Greek and Chinese ancestry; his father came from Cyprus, his mother from Singapore. Demis and his family moved frequently as his father pursued a variety of business and creative ventures. Demis was four years old when he saw his father and an uncle playing chess and asked them to teach him the game. He took to it quickly and was soon beating both of them. He showed a precocious aptitude for all games employing logic and strategy. Given his first computer at age eight, he programmed it to play the board game Othello. His fascination with both games and computers grew with every year. By age 13, Hassabis was a recognized chess master and was soon playing adults in international competition. Although he enjoyed the intellectual stimulation of chess competition, he longed to apply his skills to a larger area and decided to pursue the study of artificial intelligence (AI). At 17, he joined the computer games company Bullfrog Productions, where he worked as a designer on the game Syndicate and was lead programmer for the highly influential Theme Park. A bestseller, it won the industry’s Golden Joystick Award and spawned a host of management simulation games.
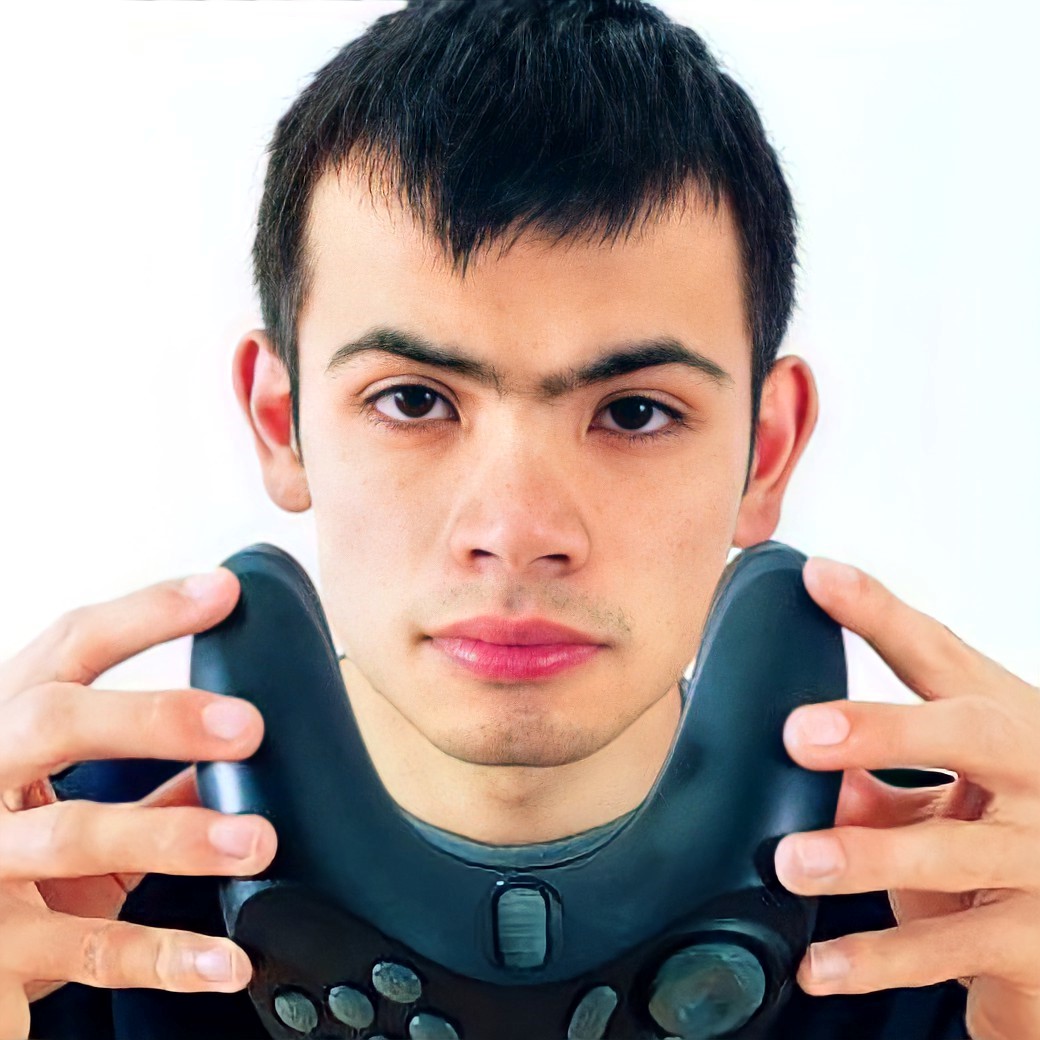
Hassabis attended Cambridge University, where he led the chess team in 1995 and 1996, and again in 1997. Graduating that year with top honors in computer science, he joined Lionhead Studios, where he was the lead programmer for the game Black & White. The following year, he founded his own company, Elixir Studios. His first game as executive designer at Elixir was Republic: The Revolution, an innovative political simulation game. It was followed by the acclaimed super-villain simulator Evil Genius, and Hassabis concluded lucrative publishing deals with Vivendi and Microsoft. While running Elixir, Hassabis continued to participate in international games competition. In the London Mind Sports Olympiad, he emerged as the winner of the Pentamind World Championship in five consecutive years, from 1998 to 2003. In 2003 and in 2004, Hassabis won the championship in Decamentathlon. In 2004 he was the world team champion in the game Diplomacy. He was also a highly successful competitor in six different seasons of the World Series of Poker.
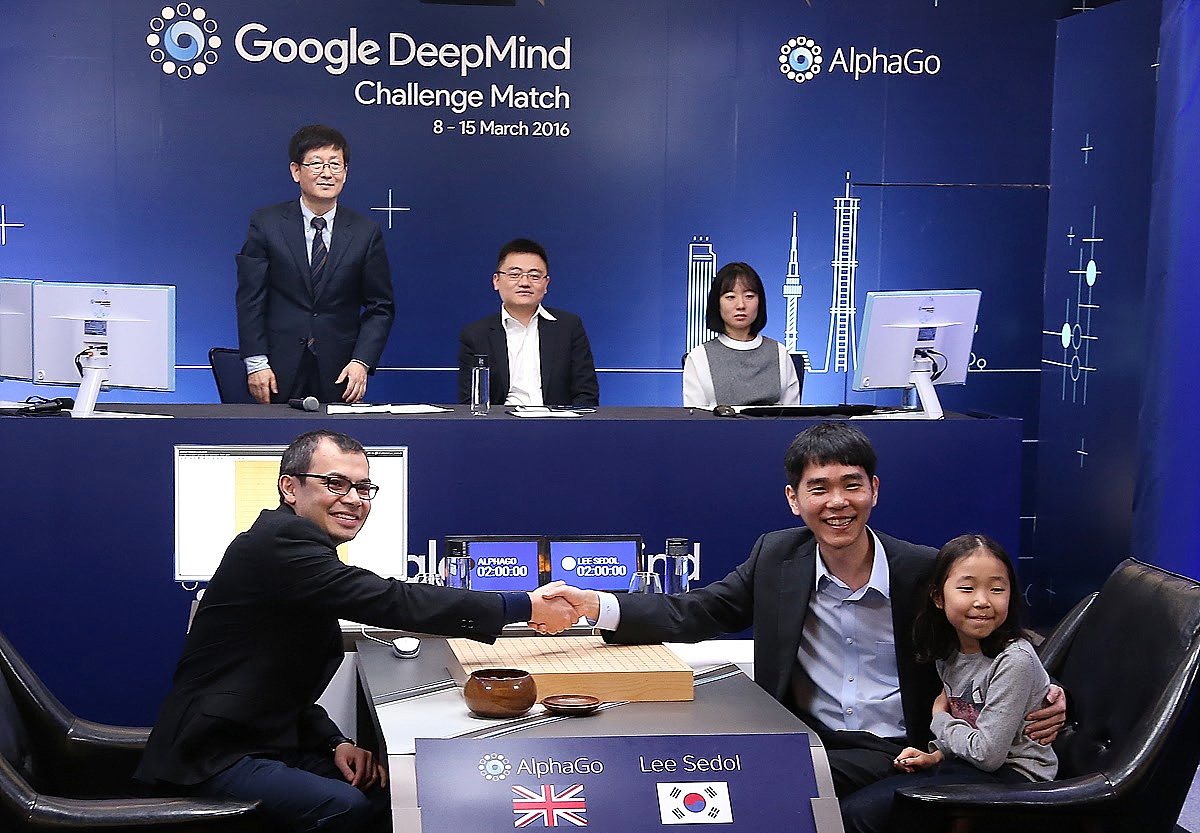
Having proven himself in world games competition and the computer games industry, Hassabis decided to study the working of the human brain to gain further insight into the possibilities of artificial intelligence. In 2005, he sold the intellectual property and technology rights to the games he had created and liquidated Elixir Studios. He began studies of cognitive neuroscience at University College London (UCL), completing his Ph.D. in 2009. He then traveled to the United States to pursue postdoctoral fellowships at Harvard and the Massachusetts Institute of Technology (MIT). He was a Wellcome Fellow at the UCL Neuroscience Unit.
His doctoral studies focused on the field of autobiographical memory and amnesia. He has co-authored papers on the subject, published in Nature, Science, Neuron, and Proceedings of the National Academy of Sciences (PNAS). His paper in PNAS established for the first time that injury to the hippocampus area of the brain — which causes amnesia — also impairs the patient’s ability to imagine other situations. Hassabis had demonstrated the neurological connection between the functions of imagination and episodic memory — both require the ability to construct a scene mentally. His achievement was cited as one of the “Top 10 Scientific Breakthroughs of the Year” by the journal Science.
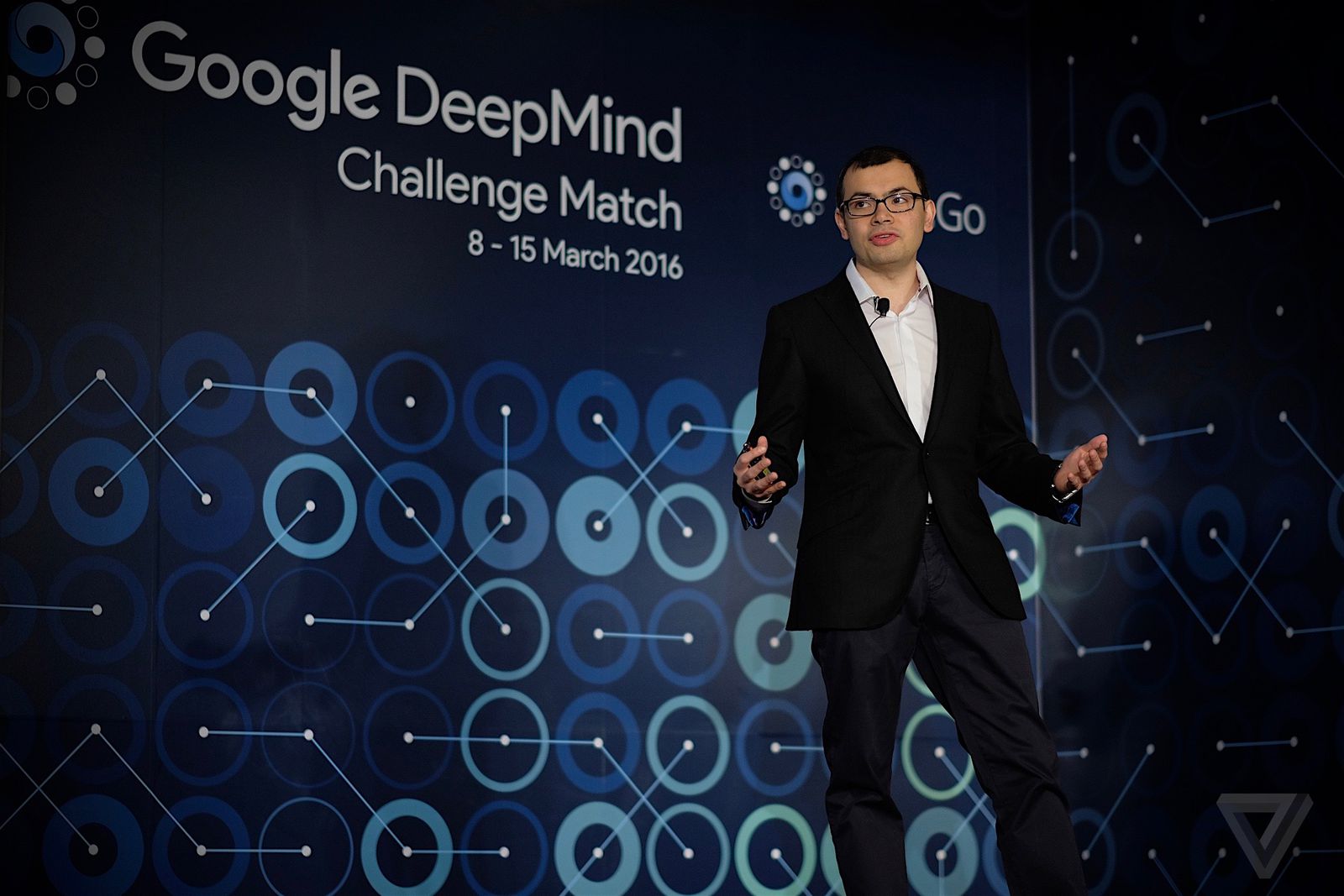
In 2011, Hassabis founded the AI company DeepMind Technologies. He defined its mission as solving “the problem of intelligence” and then using artificial intelligence “to solve everything else.” Combining insights from neuroscience and machine learning with the latest developments in computer hardware, Hassabis is seeking to construct a mechanism for general-purpose learning — “artificial general intelligence” (AGI).
Hassabis and his DeepMind colleagues focused initially on creating learning algorithms to master games. By 2013, they had created an algorithm called Deep Q-Network (DQN) that could play computer games ”at a superhuman level.” With no input other than the pixels visible on the screen, and no directions other than “achieve the maximum score,” DQN became the world’s best player of Space Invaders within 30 minutes of its introduction to the game. DeepMind’s research caught the attention of technology giant Google, which purchased DeepMind for over $6500 million in 2014. Hassabis remains CEO of DeepMind, which operates as an independently managed entity, with headquarters in North London.
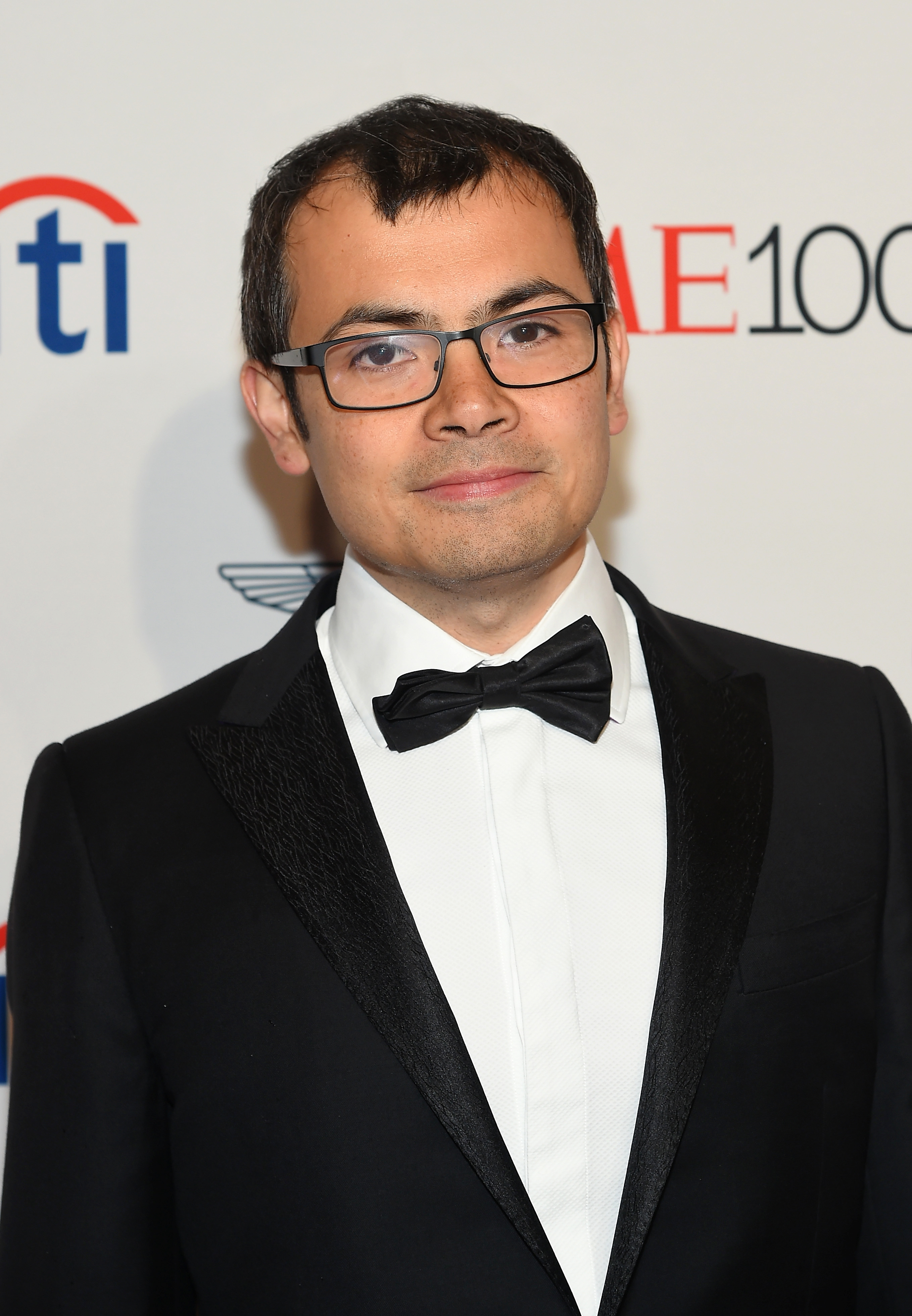
Hassabis turned his attention to the challenge posed by the ancient Chinese game Go. The multiplicity of choices available to a Go player make it virtually impossible for even a master player of the game to explain completely the logic informing a given move. Hassabis regarded the game as an ideal challenge for a learning machine. In 2015, the DeepMind program AlphaGo defeated the European Go champion with a score of 5-0. The following year, it beat a former world champion, 4 -1.
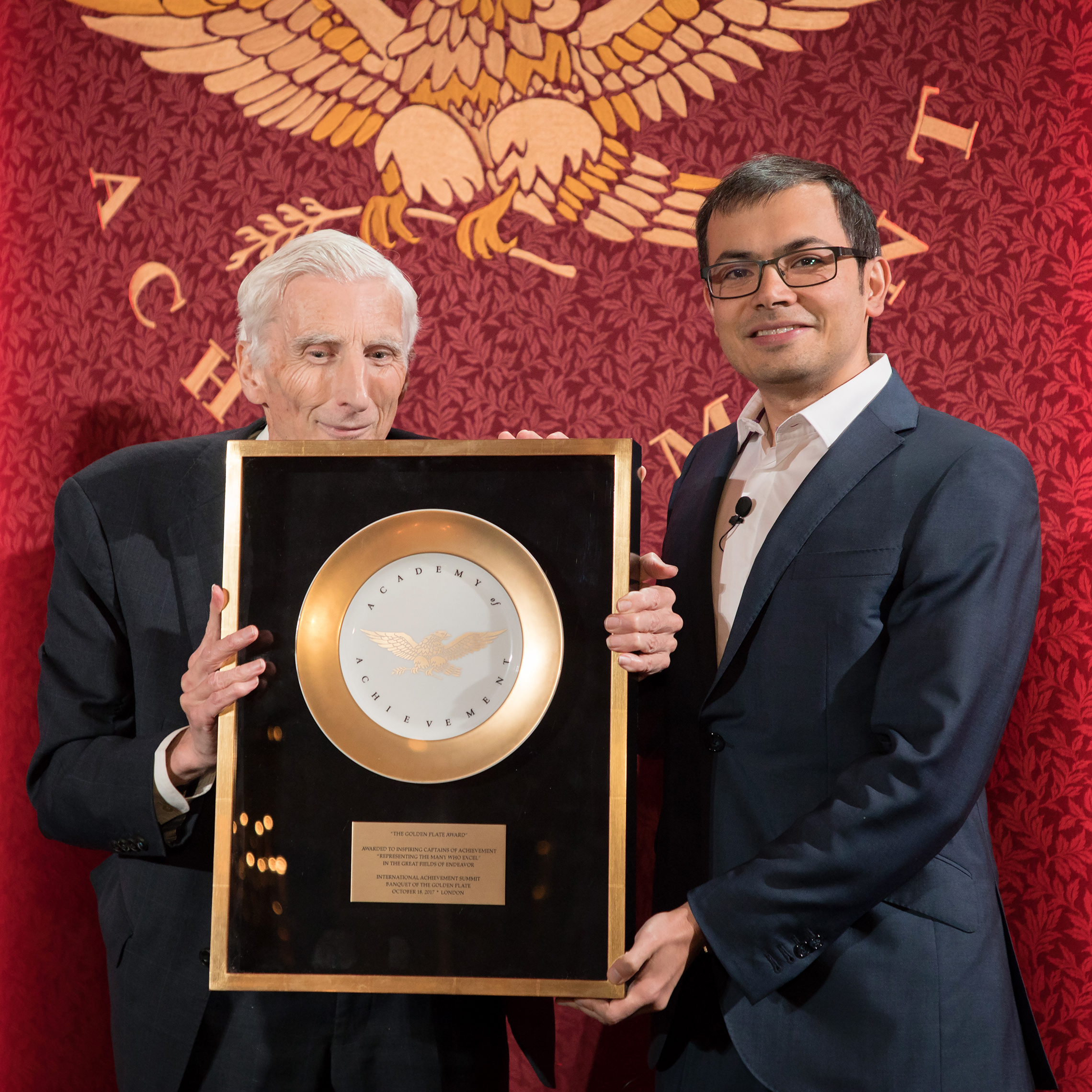
DeepMind has also produced a “neural Turing machine,” that is, a recurrent neural network model combining the fuzzy pattern-matching capabilities of an artificial neural network with the algorithmic power of a programmable computer. The company’s progress in machine learning has married the processes of deep learning and reinforcement learning to create the new field of “deep reinforcement learning.” These processes hold enormous promise for almost every field of scientific study, from medicine to astrophysics.
In 2015, the Financial Times listed Demis Hassabis as one of the “Top 50 Entrepreneurs in Europe” and the following year as the “Digital Entrepreneur of the Year.” Science magazine named AlphaGo one of the “Top 10 Scientific Breakthroughs of 2016” and TIME magazine listed Hassabis as one of the World’s 100 Most Influential People in 2017. In Britain’s 2018 New Year’s Honours, Demis Hassabis was named a Commander of the Order of the British Empire, and in May 2018, he was elected a member of the Royal Society, the world’s oldest scientific association.
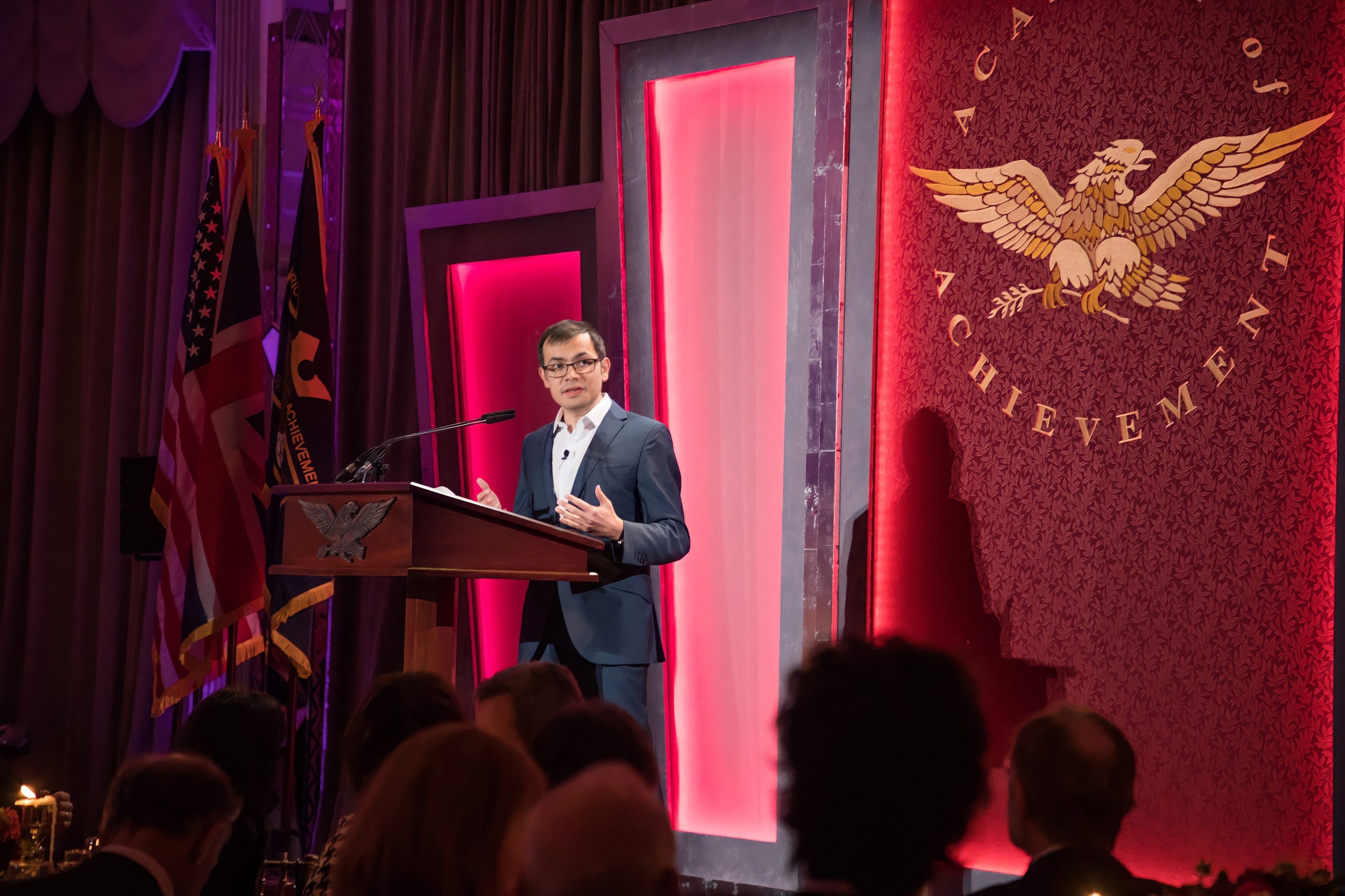
In Summer 2018, the journal Nature Medicine published a study of DeepMind’s first medical product, a new technology for analyzing OCT scans. The OCT scan (optical coherence tomography) is regularly used to detect retinal disorders, including macular degeneration and diabetes-related blindness. In an early study, DeepMind AI technology proved it can analyze OCT scans and make diagnoses with 94 percent accuracy, demonstrably better than human experts. If further trials are successful, DeepMind will make the technology available for free for the first five years. DeepMind is also working with Britain’s National Health Service to develop a mobile app to alert doctors and their patients to the threat of kidney injury.
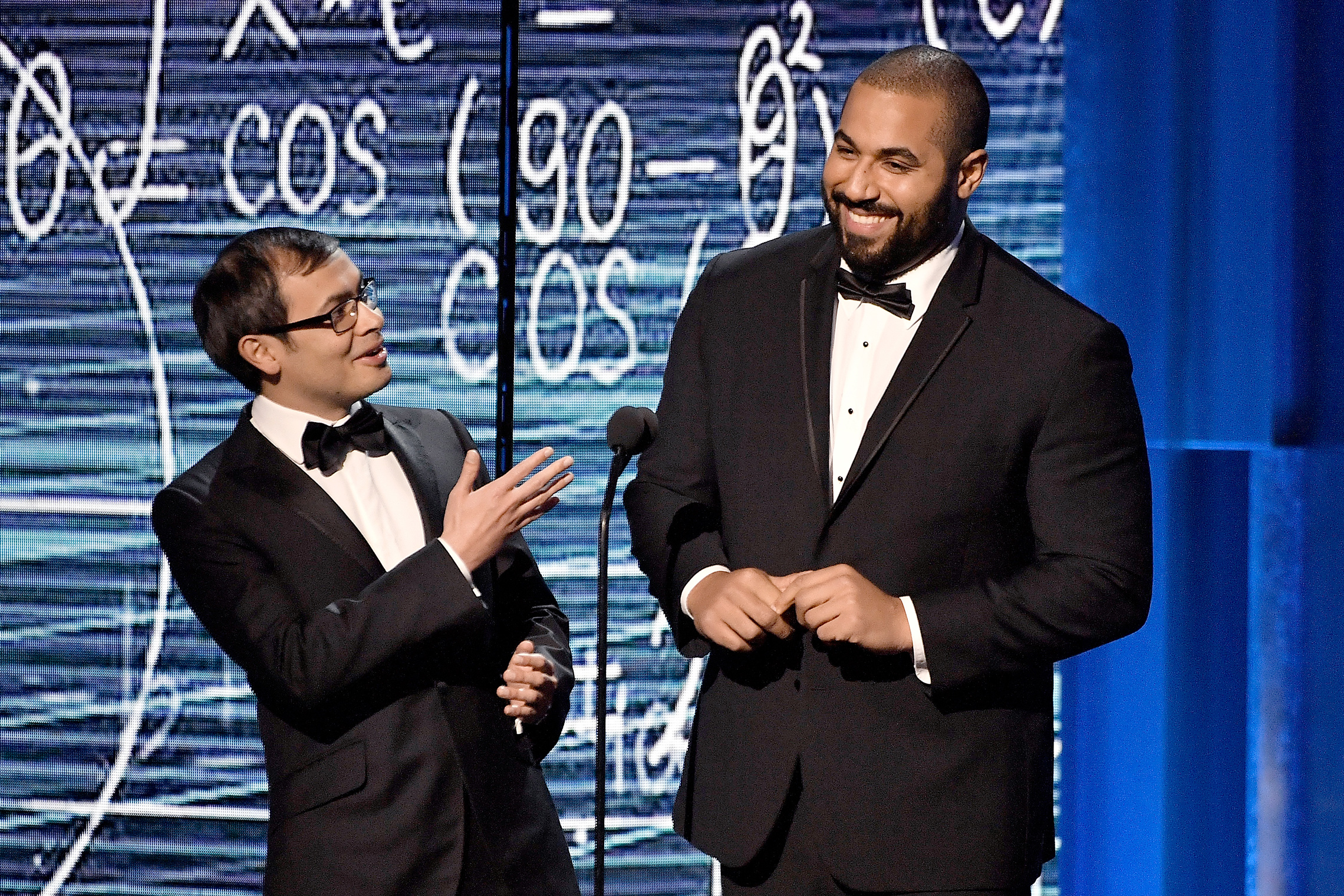
Shortly after DeepMind’s AlphaGo program defeated legendary Go player Lee Sedol, DeepMind established a small team to begin work on protein structure prediction. They developed AlphaFold, AI that can predict the shape of proteins down to the nearest atom. In December 2018, the AlphaFold program placed first in the overall rankings of the 13th Critical Assessment of Techniques for Protein Structure Prediction (CASP). In 2020, DeepMind revealed AlphaFold2 and was recognized as a solution to the 50-year “protein folding problem.” “It’s the most complex thing we’ve ever done,” says Hassabis. In July of 2021, DeepMind published a detailed description of how the system works and released the source code for free to the whole world. It also set up a public database with the European Bioinformatics Institute that it is filling with new protein structures as the AI predicts them. The database currently has around 800,000 entries, and DeepMind will add more than 100 million—nearly every protein known to science—in the next year. A handful of teams around the world have started using AlphaFold in work on antibiotic resistance, cancer, covid, and more.
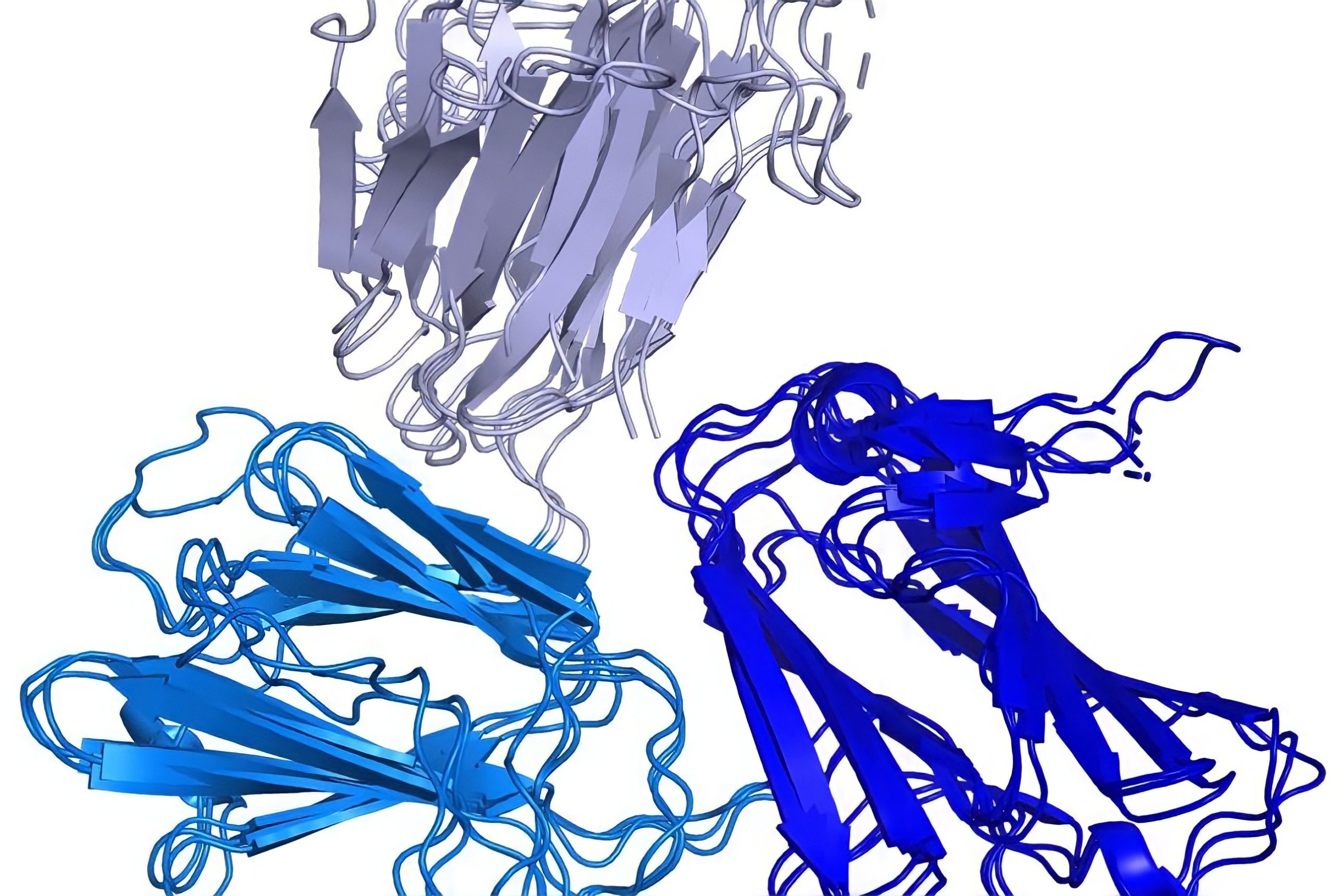
In November 2021, Hassabis announced he is now juggling his leadership at DeepMind with the CEO role at the startup Isomorphic Labs, a new sister company in Alphabet that will focus exclusively on bringing the power of AI to biotech and medicine. In July 2022, researchers at the DeepMind Technologies artificial-intelligence lab said they had predicted the structure of nearly all known proteins, a significant advance in biology that will accelerate drug discovery and help address problems such as sustainability and food insecurity. They also expanded the AlphaFold database to include 214 million predicted proteins, or nearly all of the proteins known to science. That includes all of the proteins in the human body, as well as proteins found in animals, plants, bacteria and many other organisms.
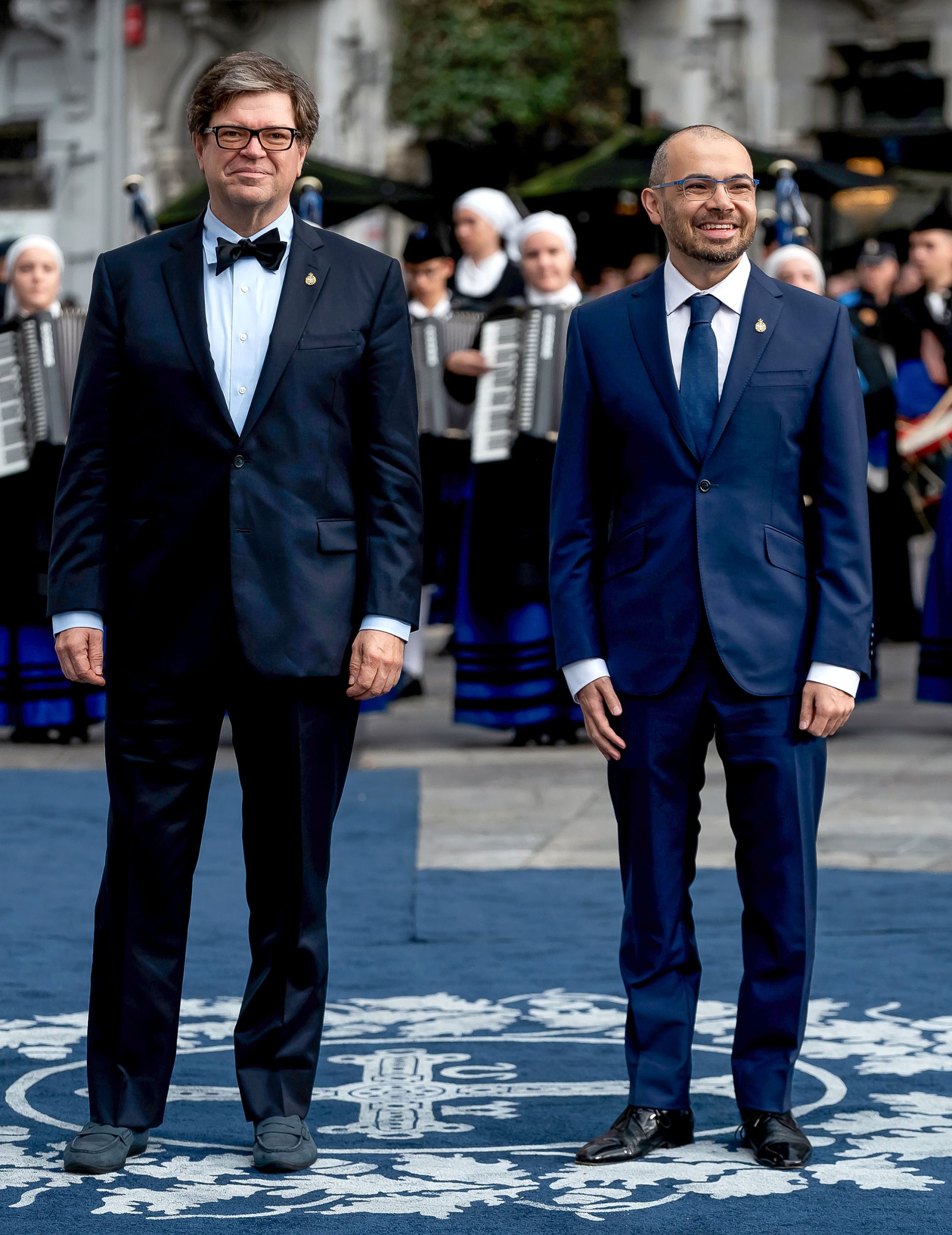
In April 2023, Alphabet announced that DeepMind would merge with their own AI lab, Google Brain. Hassabis will lead the newly formed “Google DeepMind” unit with a clear mission to develop “general AI systems” that are even more “capable and responsible”, and can be integrated into new products and services. In September 2023, the Albert Lasker Basic Medical Research Award was bestowed upon Demis Hassabis and John Jumper of Google DeepMind for the invention of AlphaFold. This groundbreaking AI tool has drastically improved the accuracy and speed of predicting 3D protein structures from amino acid sequences, deepening our comprehension of biological mechanisms and expediting drug formulation processes.
In November 2023, Google DeepMind researchers, employing the AI tool GNoME, identified 2.2 million novel crystal structures, a finding that could transform areas such as renewable energy and computation. According to a paper published in Nature, this discovery exceeds the total of all previously known stable materials by 45 times. The team intends to make 381,000 of these promising structures available for further experimentation, signifying a major leap in the speed of material discovery beyond conventional methods. This advancement dramatically broadens the range of known stable materials, opening up new avenues for technological innovation.
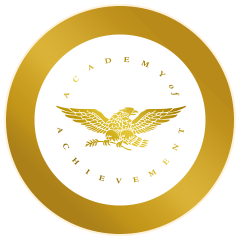
The multi-talented Demis Hassabis is a widely-cited neuroscientist, pioneering artificial intelligence researcher, award-winning game designer, successful entrepreneur, and five-time World Games Champion. A child chess prodigy, he was coding bestselling computer games while still in his teens.
He led a series of successful technology startups before returning to academia to explore the application of neuroscience to artificial intelligence. His work demonstrating the link between the functions of memory and imagination was named one of the “Top Ten Scientific Breakthroughs of 2007” by the journal Science.
Hassabis is the founder and CEO of DeepMind, a neuroscience-inspired AI company, bought by Google in 2014 — their largest European acquisition to date. At DeepMind, he has fused the fields of machine learning with the insights of neuroscience to pursue the goal of “artificial general intelligence” — AGI — a general purpose learning technology with powerful implications for every area of scientific research. DeepMind’s mission, as Hassabis puts it, is “to solve the problem of intelligence and then use intelligence to solve everything else.”
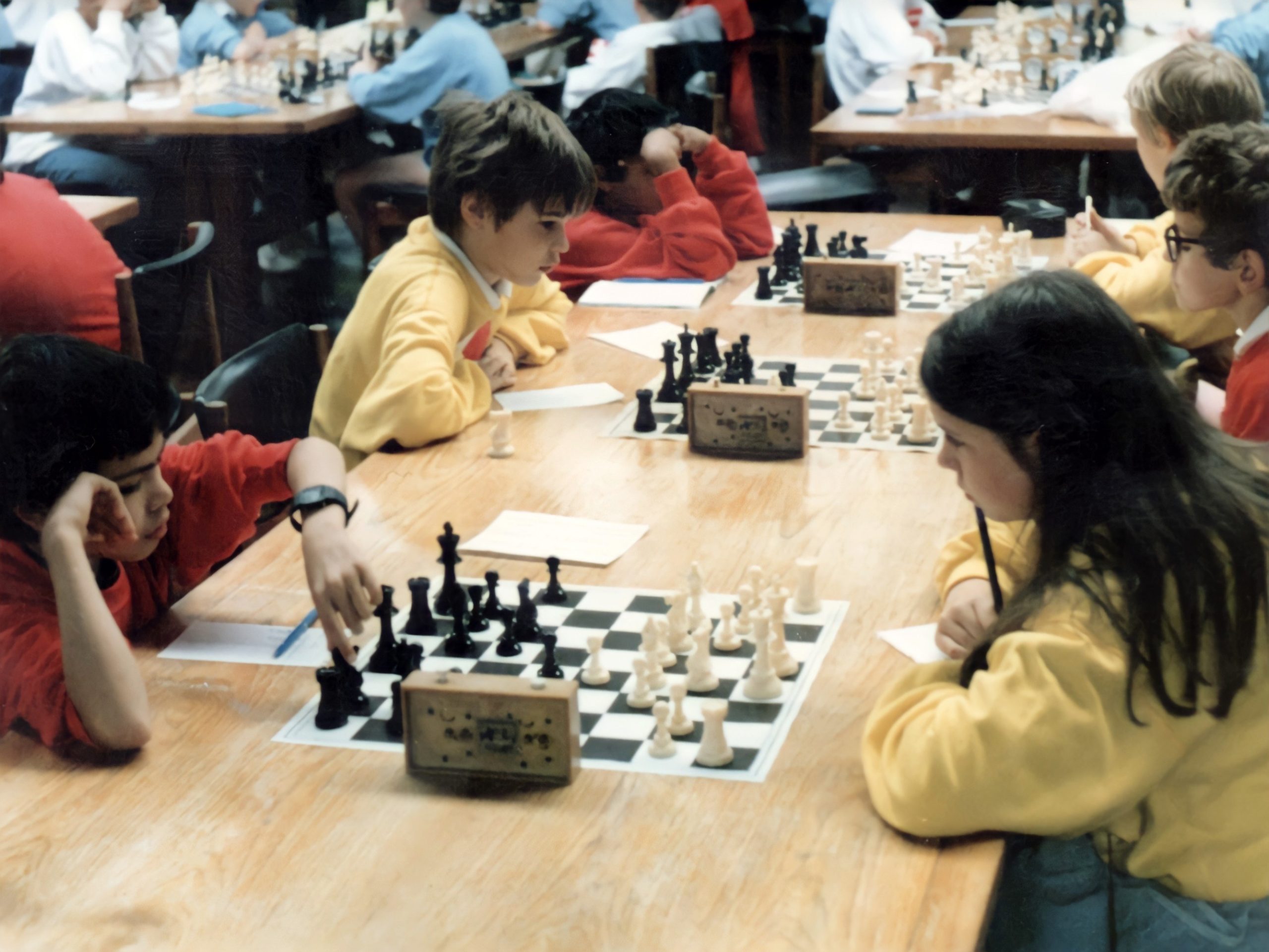
We understand you were a competitive chess player from an early age. Do you remember when you first knew you were really good at it?
Demis Hassabis: Chess is something, like, I’ve always known how to do. I learned when I was four years old. I don’t even remember learning. I’ve been told often that I saw my dad and my uncle play a game of chess, and they were just normal amateur players. Apparently, I saw them playing and I asked to play. They thought, “Well, we’ll just…” you know, humor me and just teach me the rules. And then, a couple of weeks later, I was beating both of them, and then my dad thought, “Maybe we should take him to a chess club,” and it just sort of went from there, really. And then, from all my youth, I was always captain of my age group for the national team and usually playing with much older kids. So I sort of had the chess part of me as being a big core part of my upbringing and who I am.
What do you think you learned from those years of immersion in the game?
Demis Hassabis: I think chess — actually, a number of things that I learned when I was young — it wasn’t so much the chess itself; it was the skills that you were training while you were playing chess. So problem-solving, imagination, creative thinking, strategic thinking, all of these things — if you play chess to a high level, what you’re really honing is those meta-skills. And I realized from quite young, that’s one of the reasons I liked chess was this is a great practice for me to get good — sort of like a mental gym, if you like — to get really good at those other skills, which you could then translate to all sorts of other subjects like science or business.
At age 13, you were the second-best youth player in the world, and you decided to make a change. What were you thinking?
Demis Hassabis: I had a bit of an epiphany around that age because I was playing, you know —
I was more or less a professional chess player. I was going to school. I was taking weeks off school, to go around the world playing chess, and all my summer holidays and Christmas holidays and so on. So it was pretty full on. And the rest of the time, I was studying chess. And I remember thinking that to become world champion, which was my plan at that point, then your whole life would have to be dedicated to nothing but chess and learning opening moves and theory and all this kind of stuff. And I started realizing, the more I was specializing in chess, the less it was about these meta-skills that would be useful for other things; but more, that specific knowledge would be to just chess. So it wouldn’t be useful for other things you might want to do.
And I kind of had this thought when I was in a big tournament, actually, in Liechtenstein, near Switzerland, and I remember playing a big match. It was like a ten-hour match against — I think it was the Danish champion at the time, the adult champion. And I lost the match after ten, twelve hours. And I remember thinking, “Was that really a good use of all that brain power?”
And I went for a long walk in some beautiful field — at least I remember that in my mind — in the mountains. And I remember thinking, “Maybe this isn’t — there’s this whole room full of amazingly bright people, and they’re using their minds to basically compete with each other and try and win, and what was really — maybe they should all be using their minds to solve cancer or something.” And I was just sort of thinking, “Maybe you could harness all of these bright minds. Maybe there’s a better use of that time and energy that would be better for the world.” So I made this decision, actually, at that moment, that I would move away from chess and start exploring other areas and passions of mine and use those meta-skills as my foundations for getting to these other things. But I just felt — although I loved chess, and I still love chess now — I felt it was too narrow a thing to dedicate your entire life to.
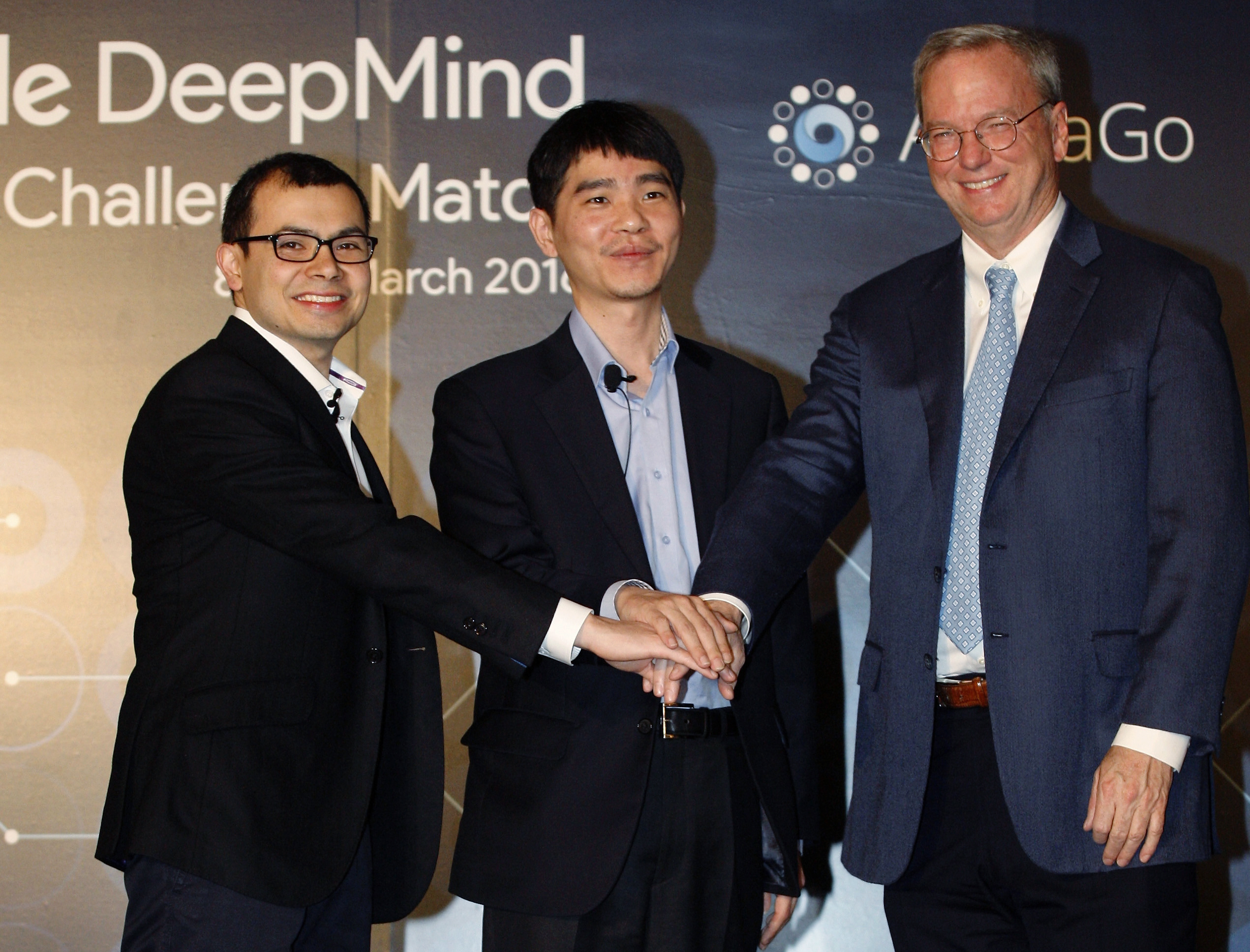
Having come to that realization, what did you dedicate your life to?
Demis Hassabis: So I dedicate my life to, you know, artificial intelligence — which is obviously what we do at DeepMind — but really, the underlying reason I work in artificial intelligence is I want to understand how the universe works. And I want to, you know — it’s the deeper scientific question. I want to try and answer the most deep questions that we have: What is consciousness? What are we doing here? What’s the universe about?
Do you have any early answers to what we’re doing here?
Demis Hassabis: I have some theories, but they’re not well-formed enough yet. I’m still working on them.
When I was a kid, I was actually — my favorite subject was physics. And usually, if you’re interested in the big questions about the universe, you would become a physicist. That’s the normal route for that. It was my favorite subject all the way up to university, but then I read this book called Dreams of a Final Theory by Steven Weinberg, who is a Nobel Prize winner, and I must have been in my late teens. And this is all about a physicist struggling to find a final theory of everything, like how everything works, and that’s what string theory’s about and so on. And I realized that they actually hadn’t got that far yet. Some of the things they were tackling were incredibly difficult, and no one seemed to be making progress. So it felt to me like maybe what was needed was more intelligence and maybe something like AI as a sort of smart tool to help scientists. It could be something that would allow us to make those kinds of breakthroughs and find the kind of theories that we were after. So it felt to me like working on AI would be more fruitful and then using that as a tool to help solve things in physics and other sciences.
What kind of breakthroughs might we see in the near future because of AI? What are you most excited about?
Demis Hassabis: The thing I’m most excited about is I think we’re on the cusp now of a new era in scientific discovery, where we’ll be able to use AI and machine learning to help us in areas of science and medicine, to make sense of enormous amounts of data that we’re generating from our experiments, and find new insights and new structure in that vast amount of data. I think that will lead to new scientific theories and new scientific breakthroughs.
How about medicine? Can you give us some examples of how AI could make us healthier?
Demis Hassabis: The obvious thing that’s already happening today has to do with radiography. So the low-hanging fruit, in some sense, is making sense of imaging data, whether that’s a PET scan of cancerous tissue or what we’ve worked directly on, which is retina scans and detecting macular degeneration very, very early on. There aren’t enough radiographers, and often the diagnosis from these scans is one of the bottlenecks to you getting treatment, and in a lot of these cases delayed treatment can be very critical to the outcome. So we’re already collaborating with many hospitals in the UK about adding in machine learning and AI tools to help with those pattern recognitions, if you like, those assessments about what’s happening in those scans. So I think that’s going to result in much more efficient diagnosis and much more efficient treatment. So I think that’s just the beginning. Then the next step will be things like drug discovery, where you’ve got some target, some virus or some bacteria, and you need to generate some new compound that will target that. I think, again there, we’re on the cusp of being able to use things like machine learning to discover new types of drugs.
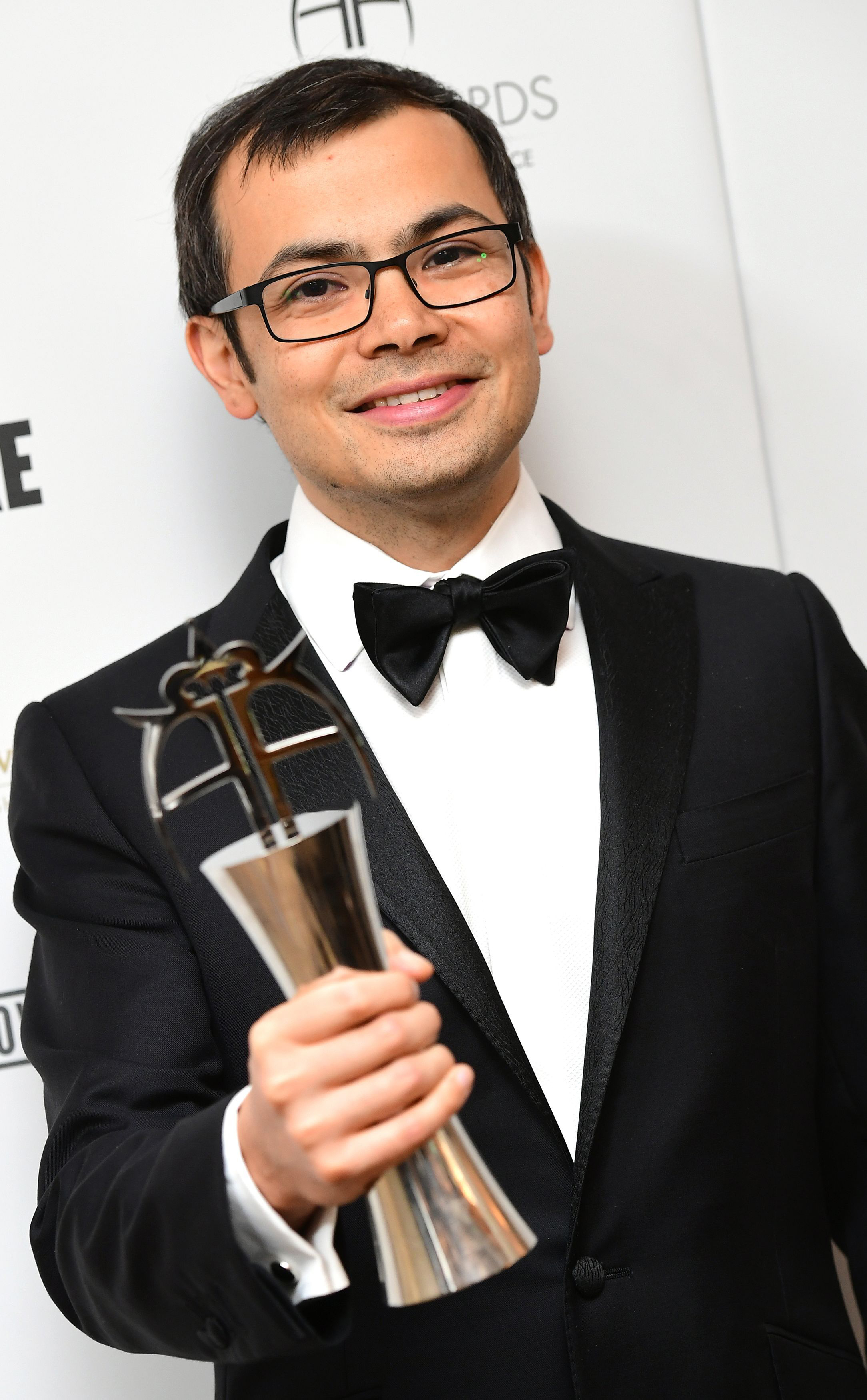
How can a machine help us with drug discovery? Can you walk us through an example?
Demis Hassabis: What happens is, you know, if you think about — it’s a massive combinatorial problem. Like you’ve got lots and lots of possibilities. So you’ve got all these molecules that you could put together to create a new compound, which would create a new drug. And then you’ve got this complex new virus that you’ve just discovered, and you need to build a compound that will attach to it, right? So, really, the problem is that we know quite a lot about physics; we know a lot about chemistry and a lot about biology; but the combinations of possibilities is huge, right? It’s astronomical, the number of compounds you can combine together. And you would need to test that — each one — laboriously. Maybe you’d have some theories about things that could work, but you wouldn’t know for sure, as a scientist. What the kinds of systems we’re building — like AlphaGo and AlphaZero, our new systems — is you can think of them as optimization programs. You give them some goal to optimize, whether that’s efficacy with a drug or winning a game, and they figure out for themselves the right search path through this massive combinatorial space. And they do that by having to be able to experience many — look at much more data than any human could comprehend — and do that far more quickly. So these systems can find patterns that are there in the data that we, just as human scientists or human experts or human medical practitioners, wouldn’t have the bandwidth to do.
We know protein folding is key for Alzheimer’s research. Could you tell us a little more about that?
Demis Hassabis: Biologists would love to know — if you give them an amino acid sequence — they would love to know what the 3D structure of that ends up folding into because the 3D structure governs the protein’s functionality. So the problem is there’s no known theory or mapping for the 2D string of amino acid sequence to the 3D structure. So what we’d like to do is build a way of predicting the 3D structure just from the 2D amino acid sequence. And the way they do it at the moment is very laborious. You have to crystallize the protein, and then you can investigate its 3D structure, but it takes years for each protein and there’s millions of proteins. So we need to somehow speed that up.
So the goal is to figure out how proteins fold. And obviously, in Alzheimer’s, one of the hypotheses is that the amyloid beta protein is misfolding and not folding in the correct way, and they don’t know why. Something’s triggering that. So I imagine it could be helpful for that, down the line. I mean it wouldn’t directly solve Alzheimer’s, but it would be something you could use as a tool to help you speed up maybe trials for drugs for Alzheimer’s, but that would be for Alzheimer’s researchers to figure out. But we’re trying to build this sort of generic platform that many different biologists could use.
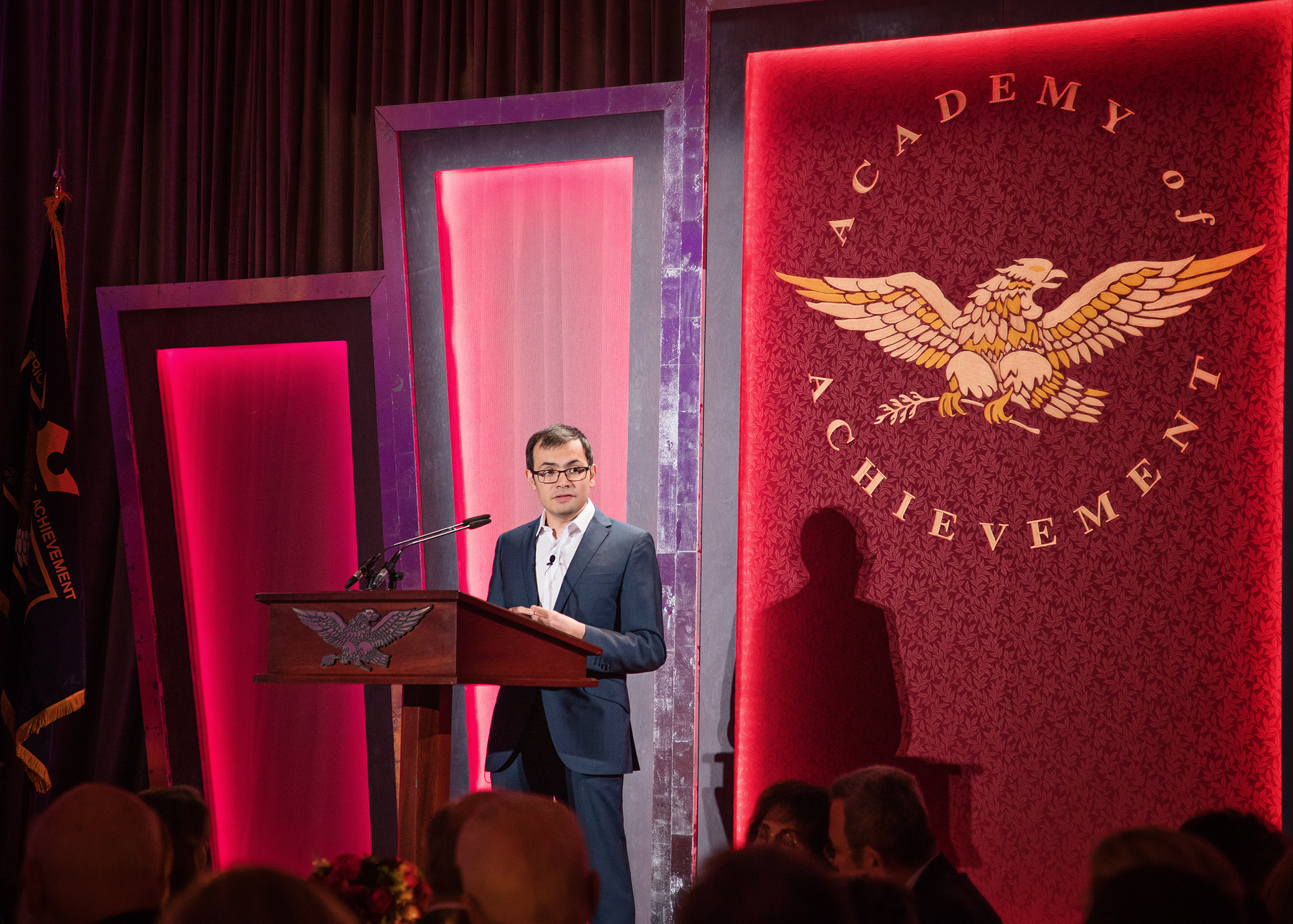
It sounds like you’re particularly interested in medicine. Is that fair to say about your goals for AI?
Demis Hassabis: We talked a lot about medicine, but I’m very interested in helping astronomers. Some people already use machine learning to discover new planets from data they have from their telescopes and find even smaller planets — exoplanets — that they couldn’t see or figure out from using their own maths. I’m interested in, you know — I’ve given a talk at CERN (European Center for Nuclear Research), and they now use machine learning to try and detect new particles. There are so many areas of science where — I mean science today is all about creating huge amounts of data from the experiments and then trying to make sense of that data. And that’s what AI does. AI — in a kind of most abstract sense — converts, you know, masses of data into actionable knowledge, right? So that’s got to be useful for all sorts of areas of science.
You’ve had such an extraordinary career. Could tell us about one “aha!” moment? Like one moment when you knew you’d found something.
Demis Hassabis: I’ve had quite a lot of “aha!” moments. I guess one of the really formative ones was when I first got my first computer. I was about eight years old. It was a ZX Spectrum. I remember starting to learn to program on it and sort of learning from books. I programmed some — one of my first programs I can remember was a program to play this game called Reversi or Othello. It’s called Othello in the UK, but it’s called Reversi, I think, in the U.S. And, you know, it played it reasonably well, and it could beat my kid brother. And I remember thinking, “This is amazing, that you can create a program that — you can go to sleep afterwards, and it can carry on number crunching — and then you can wake up the next day and it solved something for you that you wanted to know the answer to when you went to sleep.” So it seemed — again, I guess I was even then obsessed with efficiency — is that it seems incredible, like a way to enhance your mind, right? So, suddenly, you can outsource problems to this computer, and it can solve certain types of things for you. To me, that just seemed like magic and a magical extension of the mind. From then, I was sort of hooked on computers and programming and then, ultimately, AI.